Machine Learning Model Deployment Done Right: Overcoming Common Pitfalls
- Choosing the right deployment environment
- Managing resource allocation for cost and performance balance
- Scaling models to handle high traffic loads efficiently
- Ensuring low-latency inference for real-time applications
- Deploying across multi-cloud or hybrid environments
- Optimizing for GPU, CPU, and edge hardware constraints
- Handling large model sizes that slow down inference
- Reducing memory and compute requirements for efficiency
- Ensuring model accuracy doesn’t degrade post-deployment
- Implementing quantization, pruning, and distillation
- Speeding up inference with TensorRT, ONNX, and optimizations
- Managing trade-offs between speed, accuracy, and cost
- Lack of CI/CD pipelines for ML model updates
- Versioning & rollback challenges for models in production
- Automating model retraining based on new data
- Integrating ML workflows with DevOps tools
- Managing multiple models across different environments
- Reducing manual effort in deployment and scaling
- Protecting models from adversarial attacks and tampering
- Ensuring secure API access and authentication mechanisms
- Implementing AI ethics to prevent biased or harmful behavior
- Managing data privacy and regulatory compliance (GDPR, HIPAA)
- Preventing unauthorized model access or IP theft
- Ensuring encrypted storage and transmission of model data
- Detecting and mitigating model drift over time
- Handling degraded model performance post-deployment
- Automating alerts and issue detection in real-time
- Identifying high-impact use cases for AI-driven automation
- Updating models without causing downtime
- Managing retraining and feedback loops efficiently
- Ensuring smooth integration with existing business applications
- Converting models into APIs or microservices for easy access
- Handling compatibility with different data sources and formats
- Supporting multiple ML frameworks (TensorFlow, PyTorch, etc.)
- Enabling cross-platform deployment (mobile, cloud, edge)
- Addressing challenges in real-time data streaming for ML models
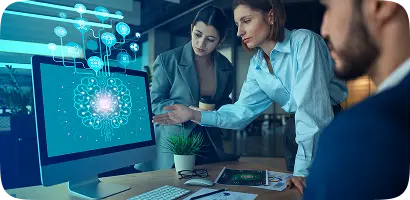
What We Do: Help you choose the best ML deployment approach for scalability and efficiency.
How We Do: Analyze your needs, recommend solutions, and design a deployment roadmap.
The Result You Get: A clear strategy for seamless, cost-effective, and future-ready ML deployment.
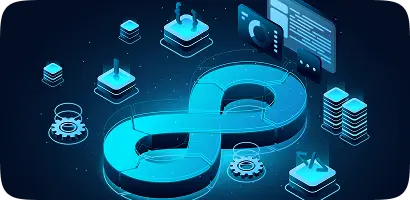
What We Do: Automate ML workflows for faster, more reliable deployments.
How We Do: Implement CI/CD pipelines, version control, and automated monitoring.
The Result You Get: Continuous updates, minimal downtime, and optimized model performance.
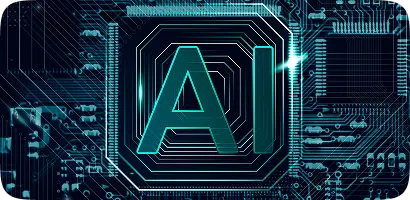
What We Do: Deploy ML models on edge devices for real-time decision-making.
How We Do It: Optimize models for low-power devices and integrate with IoT systems.
The Result You Get: Faster insights, reduced cloud costs, and smarter edge AI.
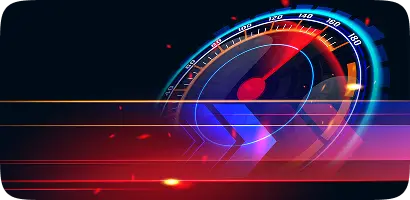
What We Do: Improve model speed, accuracy, and efficiency.
How We Do It: Apply techniques like quantization, pruning, and hardware acceleration.
The Result You Get: High-performance ML models with lower latency and cost.
The Impact of Our ML Deployment Services: What You Achieve
Deploy ML models effortlessly across cloud, on-prem, or edge environments, ensuring smooth integration with your existing systems. Our approach guarantees scalability, reliability, and minimal downtime, so your AI solutions grow with your business.
With MLOps and CI/CD automation, your models stay updated without manual intervention. Automated workflows ensure fast deployment, continuous monitoring, and effortless retraining, keeping performance at its peak.
Deploying ML models on edge devices and IoT ecosystems enables instant decision-making without relying on cloud connectivity. This results in lower latency, faster insights, and reduced operational costs for AI-powered applications.
Advanced model optimization techniques like quantization, pruning, and hardware acceleration ensure faster inference, lower resource consumption, and reduced infrastructure costs, making your AI solutions both high-performing and cost-effective.
In search of ML Deployment partner?
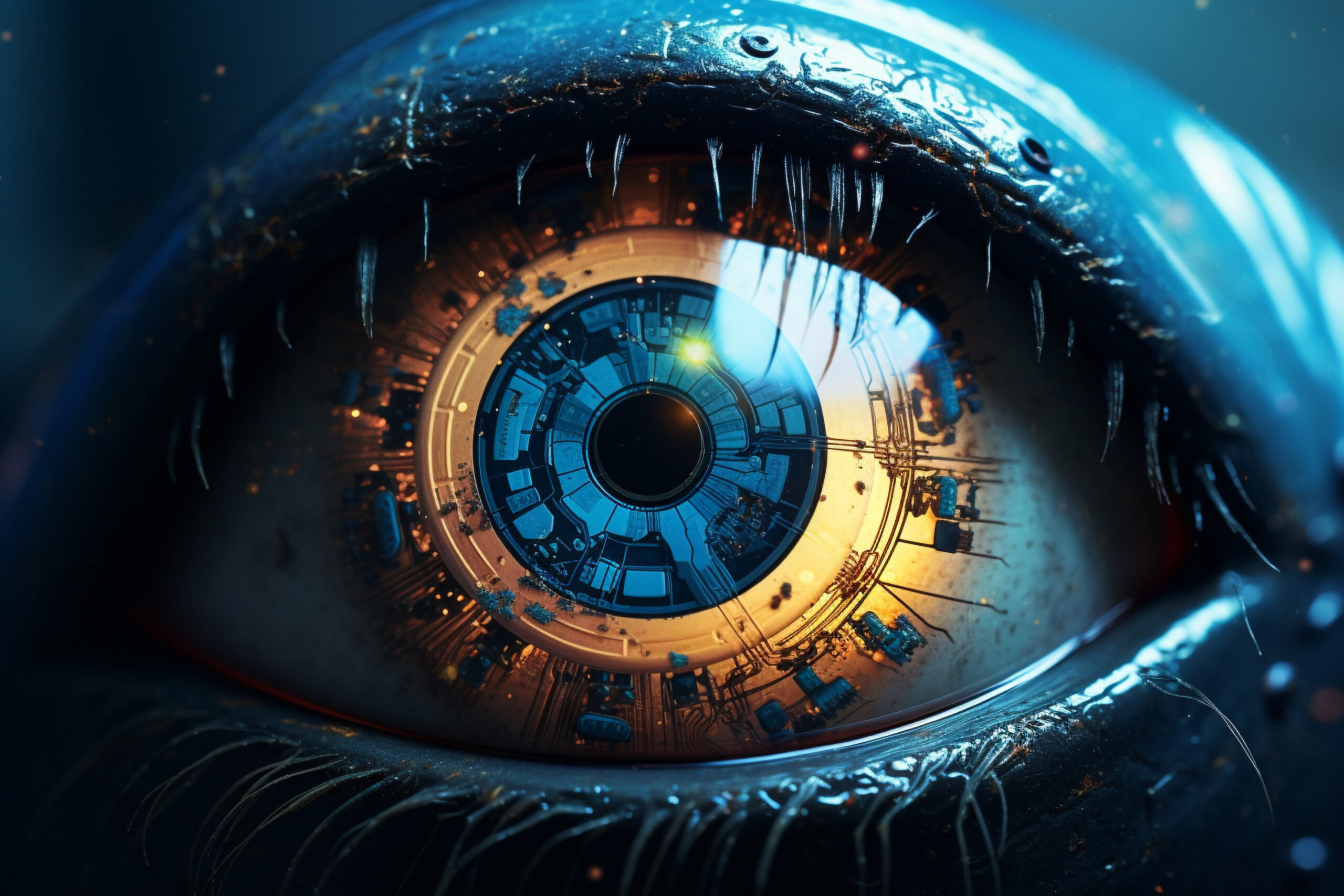
Unlimited
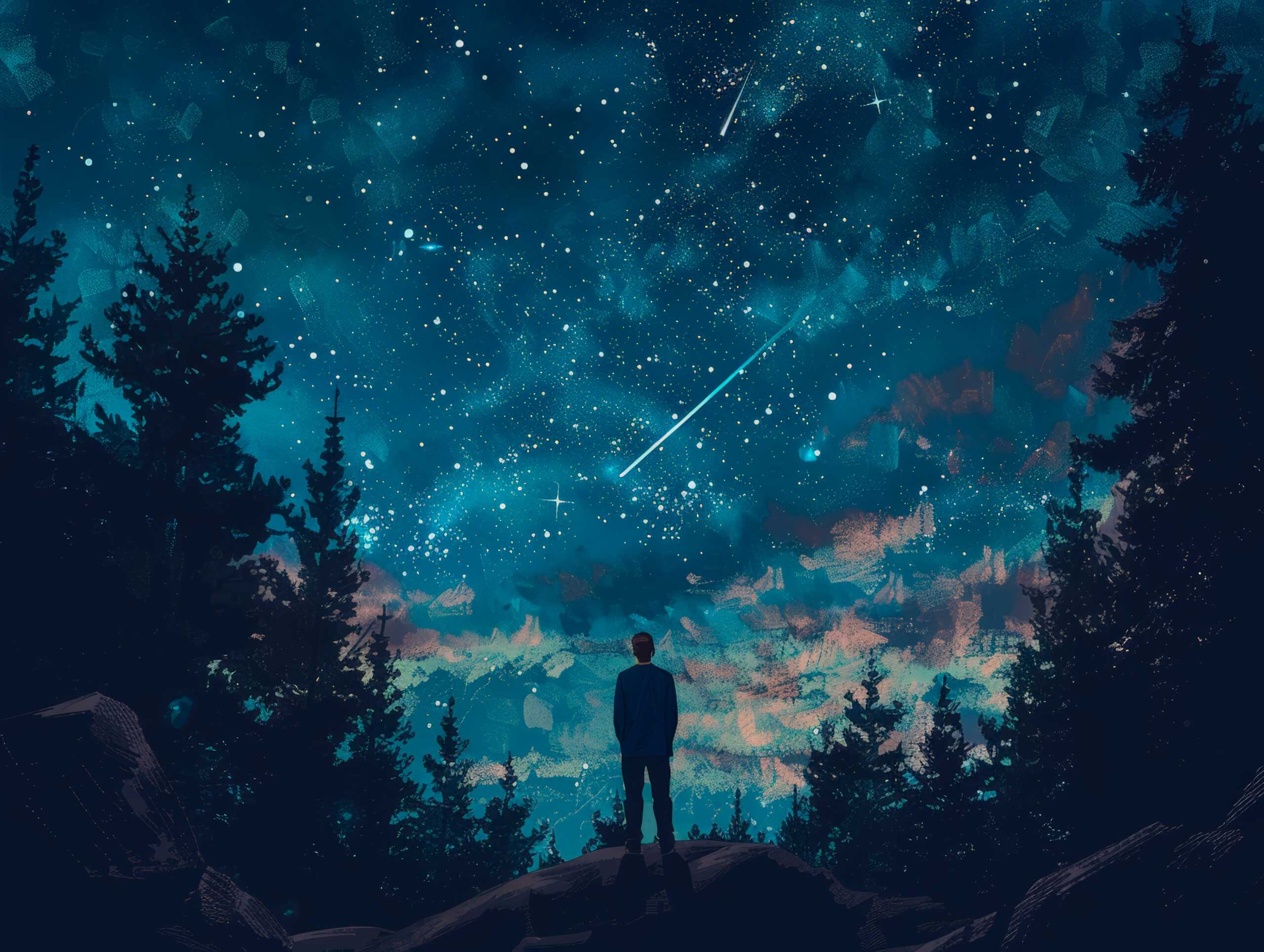
View
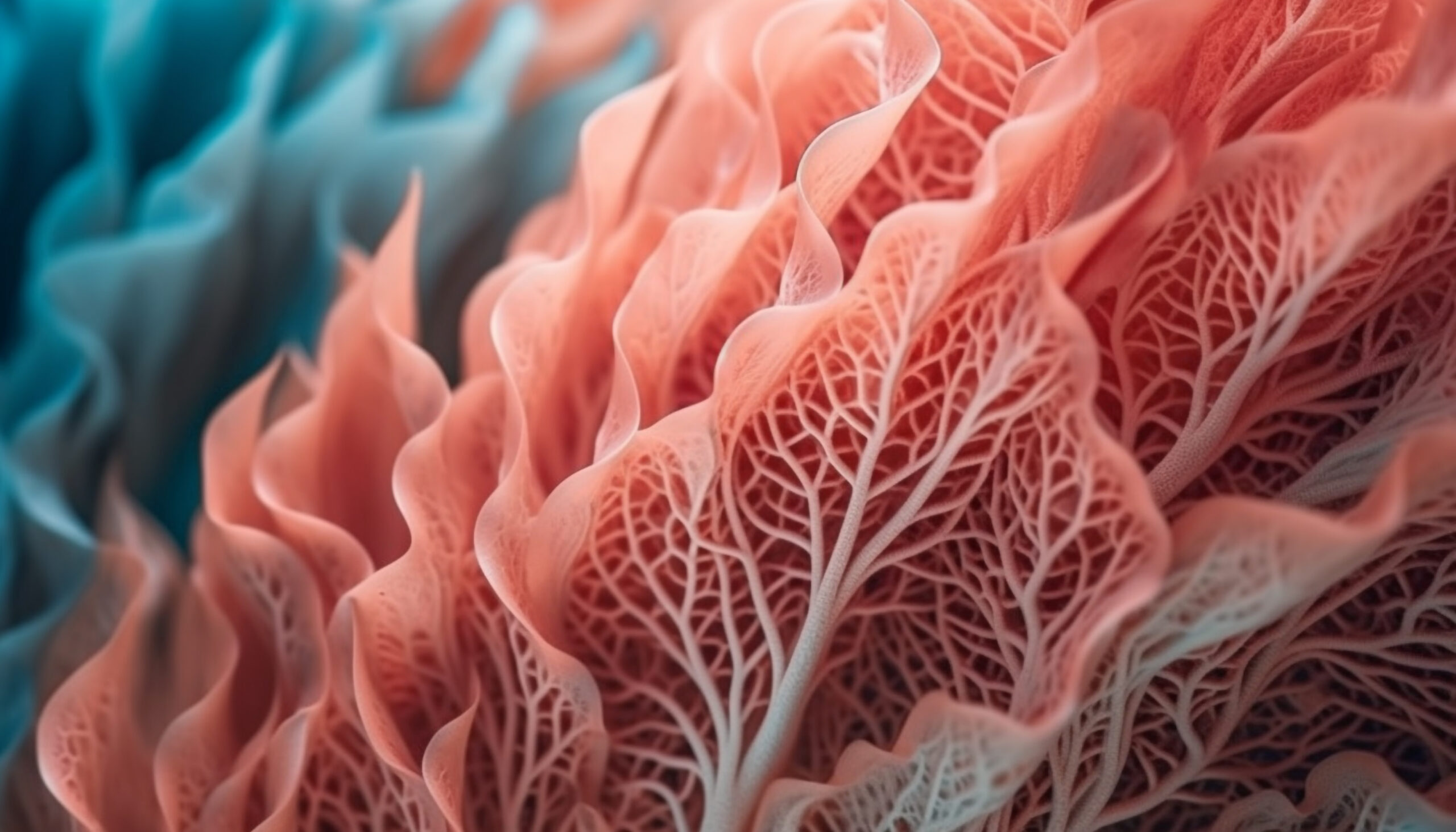
View
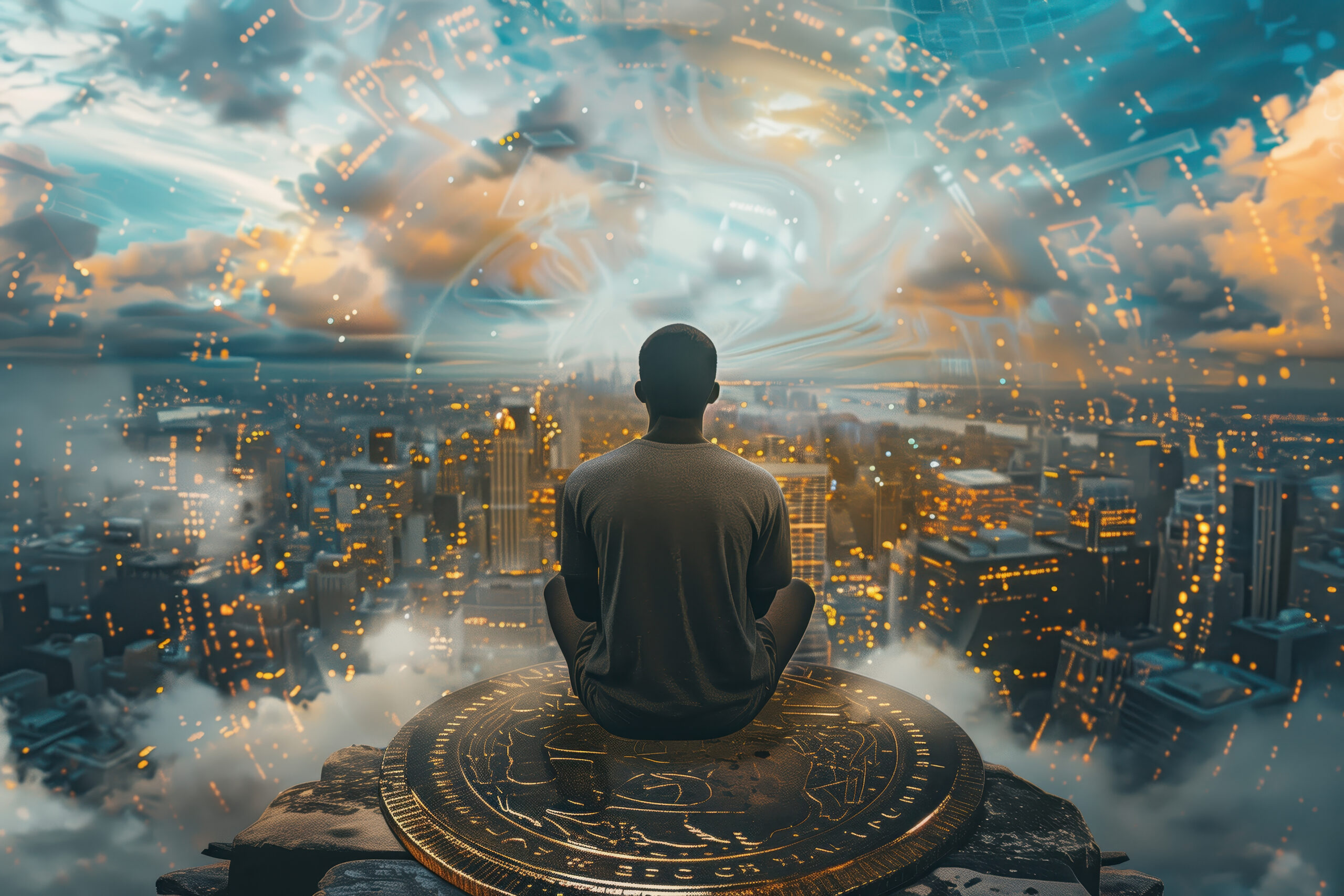
Tactics
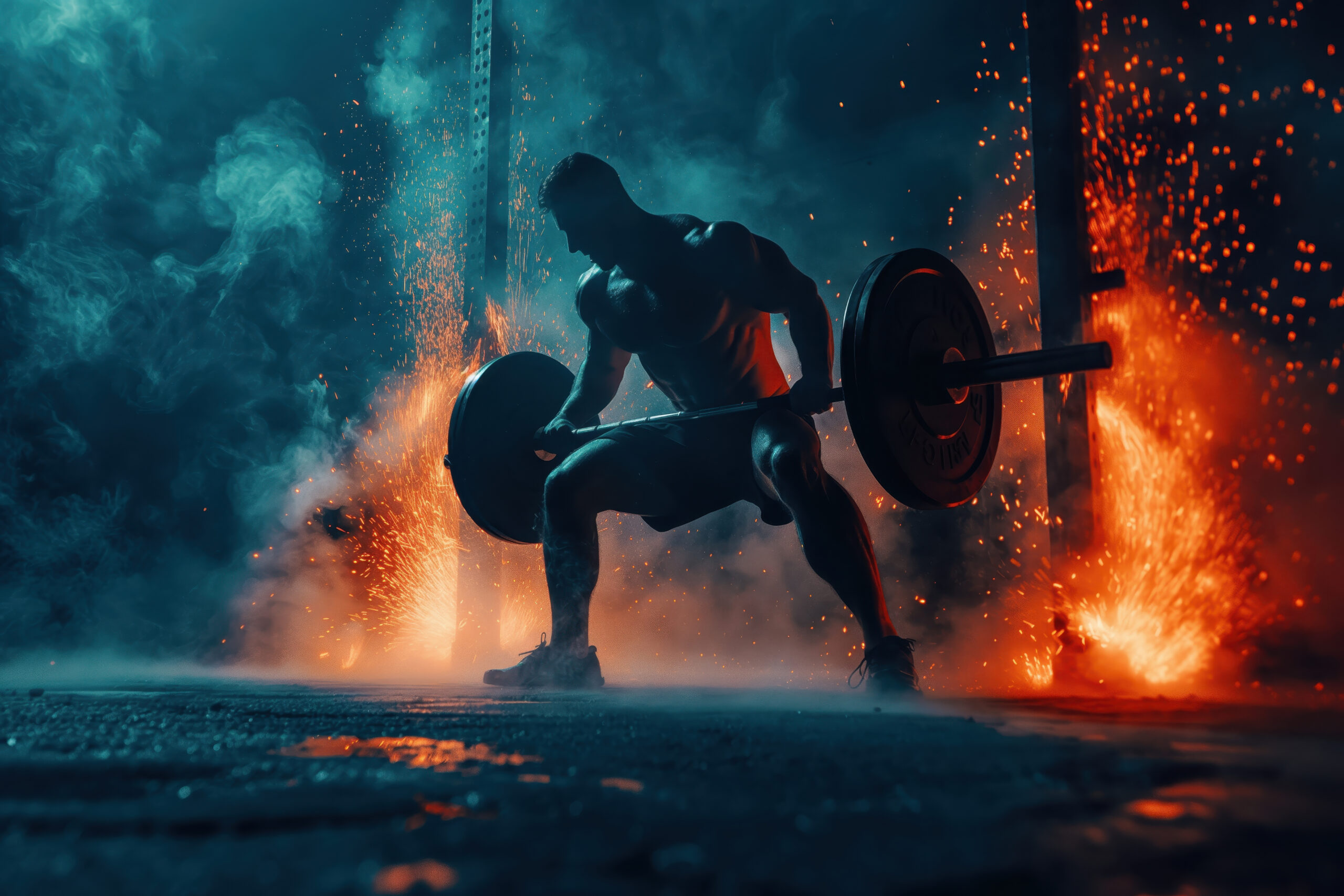
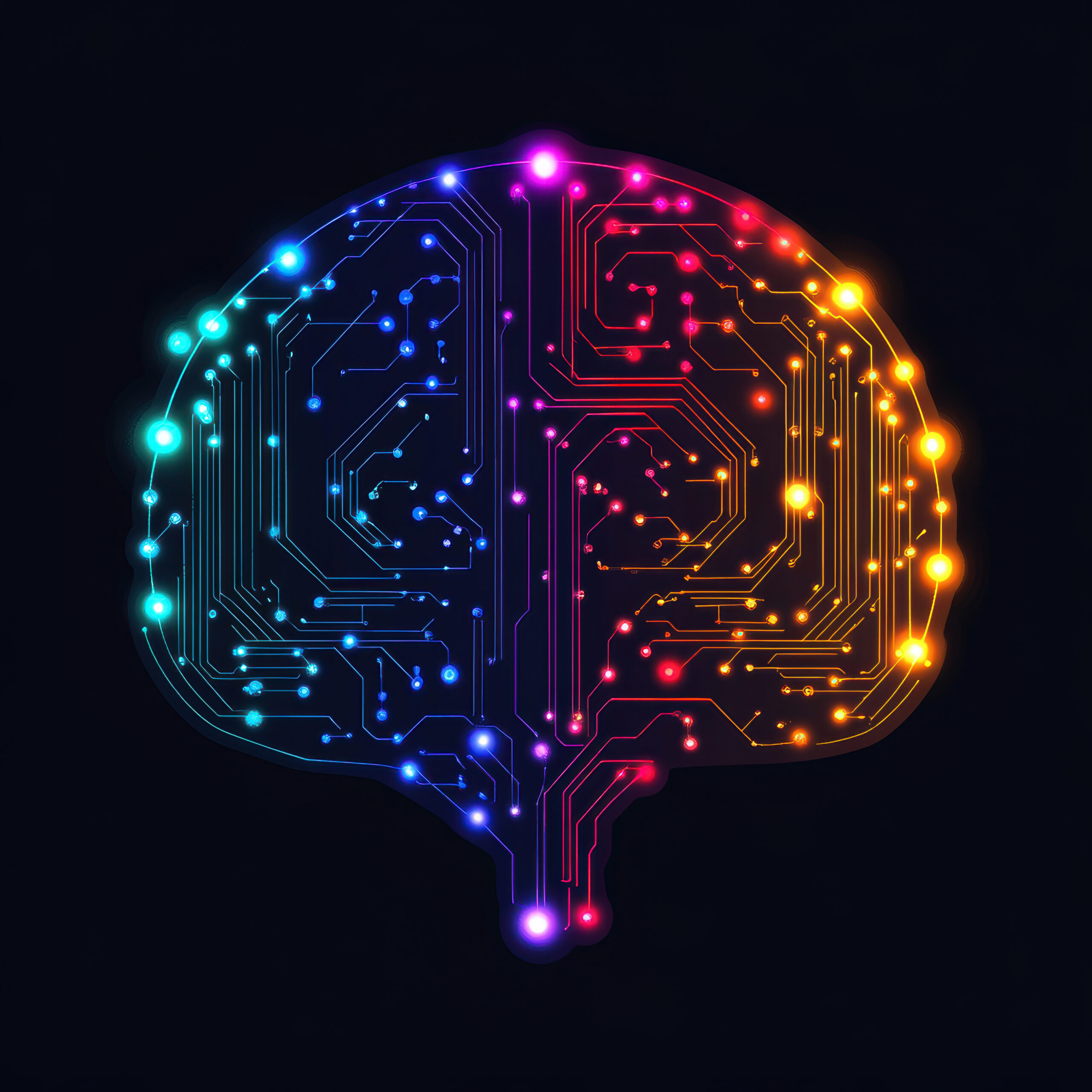
Sense
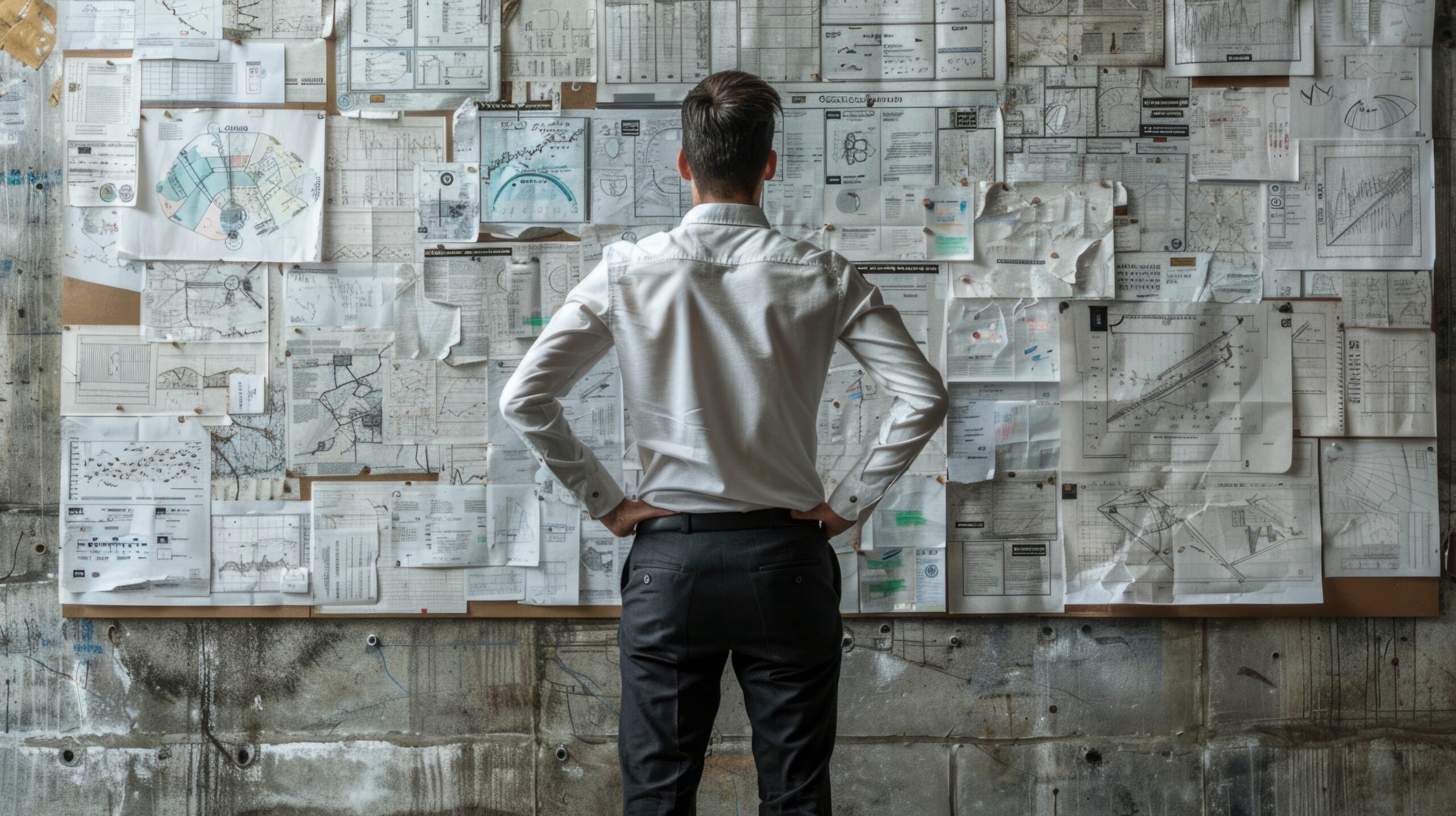
with
Problem
Statement
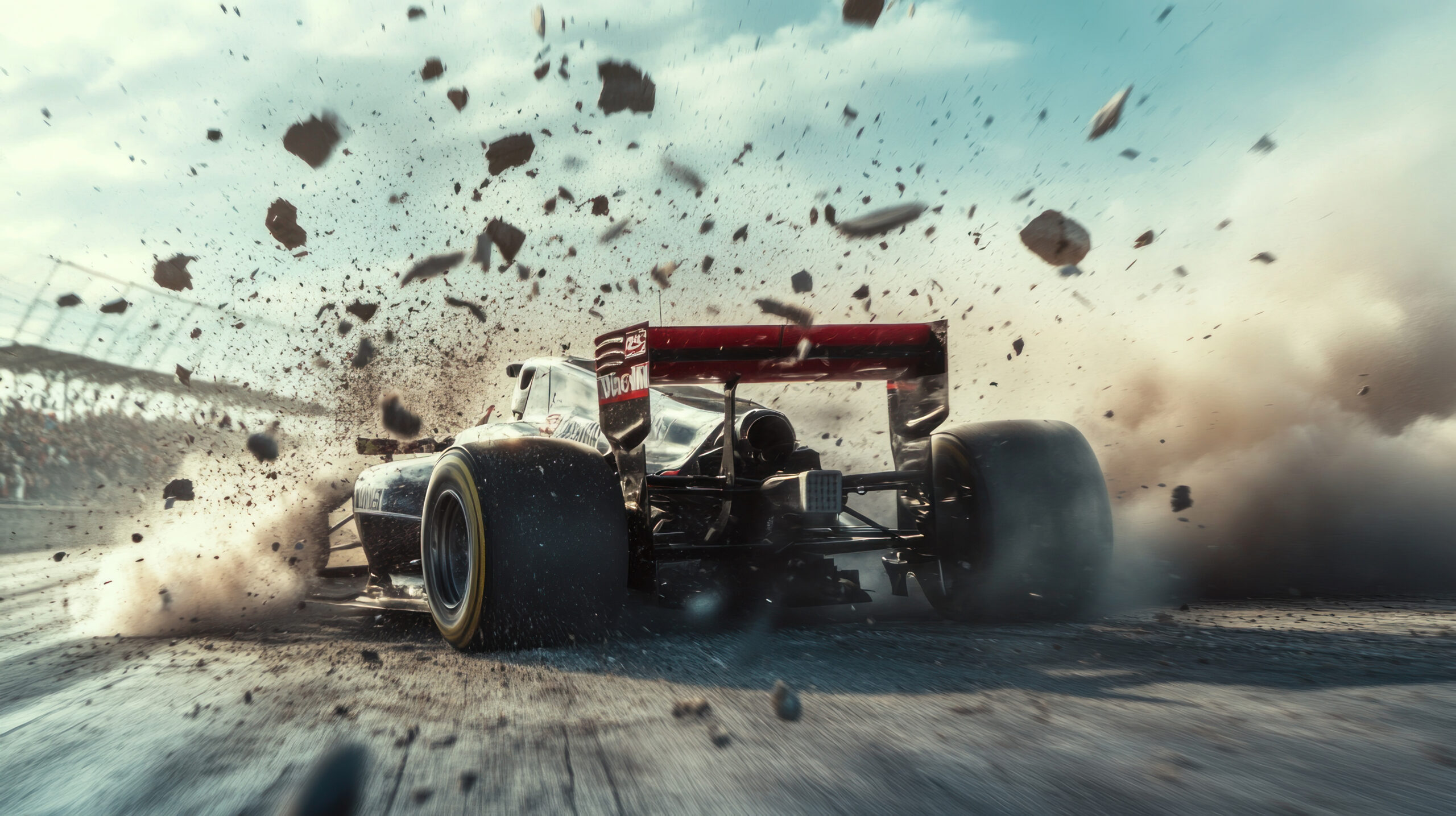
Fast
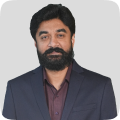
Enabling product owners to stay ahead with strategic AI and ML deployments that maximize performance and impact