We performed comparative analyses of various technologies and decided to opt for RASA Stack considering its open source nature and following advantages :
- Conversation context can be managed programmatically
- Customizable machine learning algorithms and frameworks
- Threshold confidence level can be managed at individual statements level
- Support for both supervised as well as unsupervised learning
After working over processes such as defining the use case, statistical analysis, Slack integration as channel selection, requirement of various integrations and creation of development environment, we completed the bot development briefed as below :
Data preparation or Creation of base data
This includes :
- Identifying data sources
- Aggregation, extraction and cleaning of data
- Ontology to build the vocabulary of the chat bot with industry specific terminology
Data Processing
This stage was meant for building knowledge with intent, actions and linguistic understanding. We executed it using RASA Core and RASA NLU
- Intent detection (200 types of intents can be identified so far)
- Entity detection
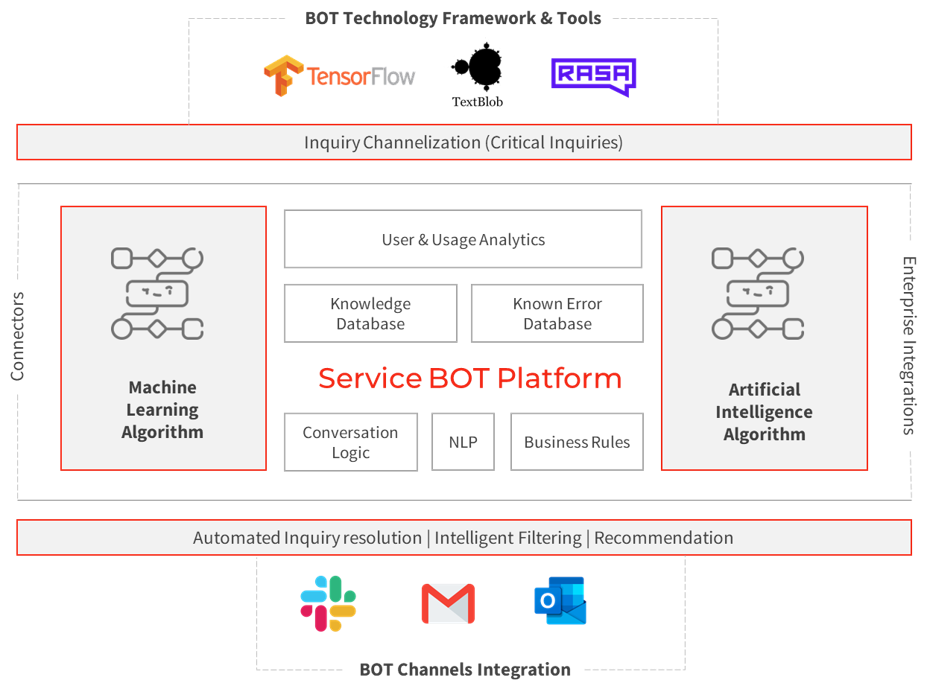
The working model of chatbot stared functioning and responding to inquiries. The bot is able to reply the questions and navigate the user to the relevant person or department, if required. Here are few of its learning capabilities :
- Direct Supervised Learning based on defined stories.
- Indirect supervised learning using generalization to new dialogues using a Machine Learning algorithm.
- Self-learning capabilities of the bot –Continuous training as interactive learning is an ongoing process to build further intelligence.
- The bot understands interactions with customers and can learn from those interactions.
- The responses are monitored and actions/ connections can be fed back to the knowledge base.
- Any known errors are flagged and fed back within the Known Error Database (KED).
- Gradually, interactive learning is initialized where in the chatbot is able to logically handle the interactions for which stories, intent, and actions are not defined.
Thus, the bot have become capable of learning, generating, predicting and recommending responses for customers.
The Email bot and Voice bot were also developed in further stages.